[ad_1]
Meta just unveiled a new AI training method that could improve how machines process information and respond to queries. Dubbed Thought Preference Optimization (TPO), this technique teaches language models to engage in internal deliberation before spitting out answers. In other words: They’re thinking, sort of.
TPO is basically like giving AI a mental pause button, allowing it to mull things over instead of blurting out the first response that comes to mind. The result? Sharper, more nuanced replies that sound less like a robot and more like a thoughtful human.
This means, TPO could bring Meta closer to offering an open source alternative to proprietary models like OpenAI’s Strawberry (aka o1), known for its complex problem-solving capabilities.
Meta’s approach differs from traditional methods like “chain-of-thought” prompting, which forces AI to show its work through different iterations. TPO keeps the mental gymnastics under wraps with the model doing everything on its own in a single shot.
The training process is also different from simply telling the model to “think step by step.” Starting with a basic instruction-following model, researchers prompt it to generate internal thoughts before answering. Through iterative reinforcement learning, the AI hones its thinking skills, guided by a judge model that evaluates only the final output—which is what the user sees.
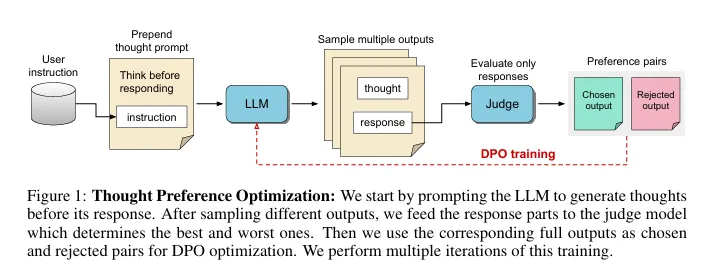
This hands-off approach allows the AI to develop its own unique thought patterns, potentially leading to more creative and adaptable problem-solving. It’s a step towards AI that doesn’t just follow rules, but actually understands the reasoning behind them.
Meta’s innovation draws inspiration from cognitive science, mimicking the human tendency to pause and reflect before tackling complex questions. If AI models learn to dedicate more “compute time” for tougher tasks, then the next generation of open source models could massively outperform the ones we are currently using.
The best part is that Meta’s TPO technique doesn’t need mountains of new data to work its magic. It builds on existing AI architectures, tweaking them to simulate a thought process without human hand-holding. This could fast-track the development of smarter AI assistants, chatbots, and other language-based tools, giving them more creativity in their approaches to problem-solving.
Meta’s researchers tested their approach against industry-standard benchmarks. TPO-trained models flexed their newfound cognitive muscles, outperforming their non-thinking counterparts on complex tasks.
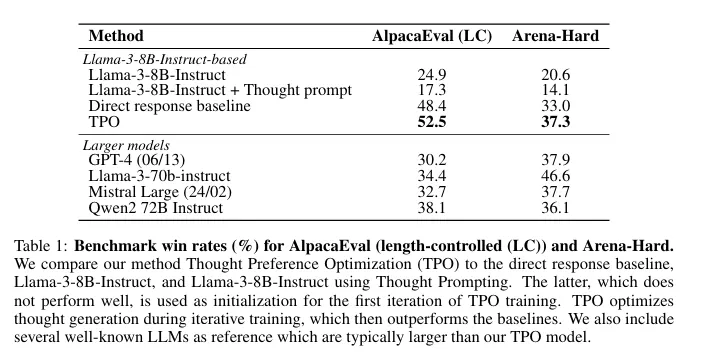
Closer to an open-source Strawberry?
Meta has been making interesting advances in the area of making AI more intelligent. Just three months ago, its researchers introduced “System 2 distillation,” a technique that teaches large language models (LLMs) how to solve complex tasks without outputting unnecessary steps.
System 2 distillation, inspired by human cognitive processes, teaches LLMs to perform complex tasks without requiring step-by-step prompting—which is usually considered the go-to approach in advanced prompt engineering. By fine-tuning models on verified responses to System 2 prompting techniques, researchers showed that AIs can internalize sophisticated reasoning skills, often matching or surpassing the performance of explicit reasoning methods.
System 1 thinking is fast, intuitive, and automatic. It’s the mental process we use for quick judgments, pattern recognition, and familiar tasks. In AI terms, this aligns with how large language models typically operate—rapidly generating responses based on learned patterns.
System 2 thinking, by contrast, is slow, deliberate, and analytical. It’s the type of processing that humans engage in for complex problem-solving, logical reasoning, and planning. AI researchers have been working to replicate this in language models through various prompting techniques that force the AI to show its work or reason step-by-step.
Meta’s Thought Preference Optimization and related research into System 2 distillation represent attempts to bridge these two modes of thinking in AI. The goal is to imbue AI models with the ability to engage in deep, System 2-style reasoning without sacrificing the speed and efficiency of System 1 processing.
This approach involves training AI to internalize complex reasoning processes. By doing so, the models can tackle intricate problems more efficiently, mimicking how humans transition from conscious, effortful thinking to more automatic processing as they gain expertise in a task.
The timing couldn’t be better, as Meta’s research comes on the heels of a tumultuous month in the open source AI space. The much-hyped Reflection 70B model, touted as a reasoning powerhouse, turned out to be smoke and mirrors. What was promised as a model with embedded chain of thought before OpenAI released o1 ended up being a model incapable of delivering on its promises, with some users even accusing the creators of simply using a wrapper on Anthropic’s Claude.
Now, its developers are pointing fingers at each other in different public postmortems, leaving the AI community reeling. Matt Schumer, the man behind the idea, is currently training a new version with his own hardware and datasets.
If Meta’s approach proves successful, then it could pave the way for an open-source rival to OpenAI’s o1 model. An open-source alternative could democratize access to this kind of advanced AI thinking.
Edited by Andrew Hayward
[ad_2]
Source link